Sentiment Analysis with NLTK: Understanding and Classifying Textual Emotion in Python
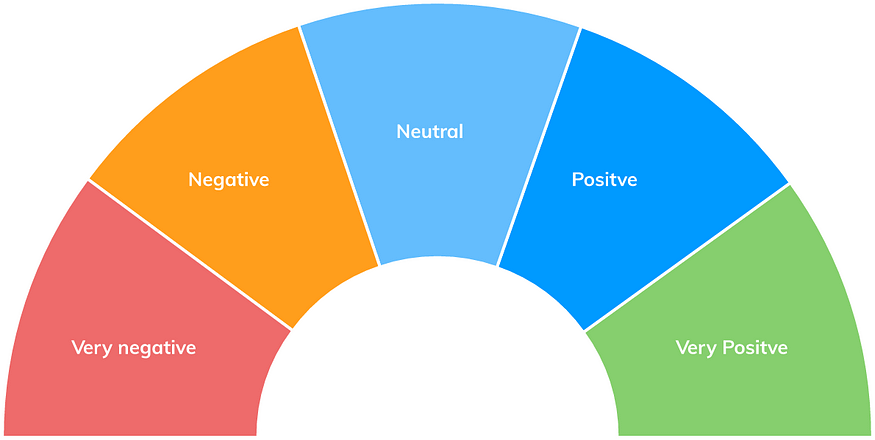
Sentiment analysis is the process of understanding and classifying emotions in textual data. With the help of natural language processing (NLP) techniques and machine learning algorithms, we can analyze large amounts of textual data to determine the sentiment behind it.
In this tutorial, we will use Python and the Natural Language Toolkit (NLTK) library to perform sentiment analysis on text data.
Sentiment Analysis with NLTK in Python
Import Libraries
We will start by importing the necessary libraries, including NLTK for NLP tasks and scikit-learn for machine learning algorithms.
import nltk
from nltk.sentiment import SentimentIntensityAnalyzer
from sklearn.metrics import accuracy_score, confusion_matrix, classification_report
Load and Prepare Data
Next, we will load and prepare the textual data for sentiment analysis.
# Load data
data = []
with open('path/to/data.txt', 'r') as f:
for line in f.readlines():
data.append(line.strip())
# Tokenize data
tokenized_data = []
for d in data:
tokens = nltk.word_tokenize(d)
tokenized_data.append(tokens)
In this example, we load the textual data from a file and tokenize it using NLTK.
Perform Sentiment Analysis
Next, we will perform sentiment analysis on the tokenized data using NLTK’s built-in SentimentIntensityAnalyzer.
# Perform sentiment analysis
sia = SentimentIntensityAnalyzer()
sentiments = []
for tokens in tokenized_data:
sentiment = sia.polarity_scores(' '.join(tokens))
if sentiment['compound'] > 0:
sentiments.append('positive')
elif sentiment['compound'] < 0:
sentiments.append('negative')
else:
sentiments.append('neutral')
In this example, we use the SentimentIntensityAnalyzer to perform sentiment analysis on each tokenized data point. We classify each data point as positive, negative, or neutral based on the compound score returned by the analyzer.
Evaluate Model Performance
Finally, we can evaluate the performance of the sentiment analysis model using accuracy, confusion matrix, and classification report.
# Evaluate model performance
labels = ['positive', 'negative', 'neutral']
y_true = ['positive' for _ in range(10)] + ['negative' for _ in range(10)] + ['neutral' for _ in range(10)]
y_pred = sentiments
accuracy = accuracy_score(y_true, y_pred)
confusion = confusion_matrix(y_true, y_pred, labels=labels)
report = classification_report(y_true, y_pred, labels=labels)
print('Accuracy:', accuracy)
print('Confusion Matrix:\n', confusion)
print('Classification Report:\n', report)
In this example, we evaluate the model performance using a sample dataset of 30 data points with equal distribution of positive, negative, and neutral sentiments. We calculate the accuracy, confusion matrix, and classification report of the sentiment analysis model.
In this tutorial, we have learned how to perform sentiment analysis on textual data using NLTK and Python. With the help of NLP techniques and machine learning algorithms, we can now analyze large amounts of textual data to understand and classify emotions.